Know thy customer
While technology keeps advancing, complexity increases as well – and so do concerns about online privacy. At least part of the solution is the customer data platform (CDP), which is useful in SMEs as well as complex, international organisations.
Tackle complexity and diversity with a customer data platform
Remember the whole ‘customer 360’ idea? Back in the early noughties, it all sounded so simple:
- Collect customer information across all touchpoints.
- Read your customers minds.
- Profit.
Fast forward to today and the overall realisation is that leveraging customer data is just not that simple. While technology keeps advancing, complexity increases as well – and so do concerns about online privacy. At least part of the solution is the customer data platform (CDP), which is useful in SMEs as well as complex, international organisations. In this article, we’ll explore how CDPs can help the latter with their specific challenges.
Gaining that much-coveted 360-view of your customers is relatively easy when you’re the village baker. But what if you’re an international company with numerous departments spread across the globe that speak different languages? Where data taxonomy – terminologies, labels and even methodologies – differs from site to site or even from team to team?
Optimising the data flow
Ensuring everyone in the company is using the same (customer) data taxonomy is one of the main prerequisites for a successful data strategy. It’s also needed to ensure that a customer data platform functions properly and is used efficiently.
This requires strict agreements, proven-yet-flexible methodologies, and a solid technological foundation. For large and complex organisations, a customer data platform helps to generate a unified customer view, where different data types from various sources are combined and unified in meaningful ways to offer relevant insights and improved reporting, and/or to fuel machine learning algorithms.
That’s quite a mouthful, solet’s first revisit the basics. To improve your customers’ experiences, you need to know who your customers are. Which data you need depends on the use case. This data can be obtained at various touch points in the sales funnel. Until recently, third-party cookies were the most common method to do so, but these will soon disappear. As a result, companies need to develop customer data strategy to convince prospects to hand over their personal information. In addition to personal information, you could also factor in external data for a more complete picture – e.g. current trends, the weather, most recent outrage, etc.
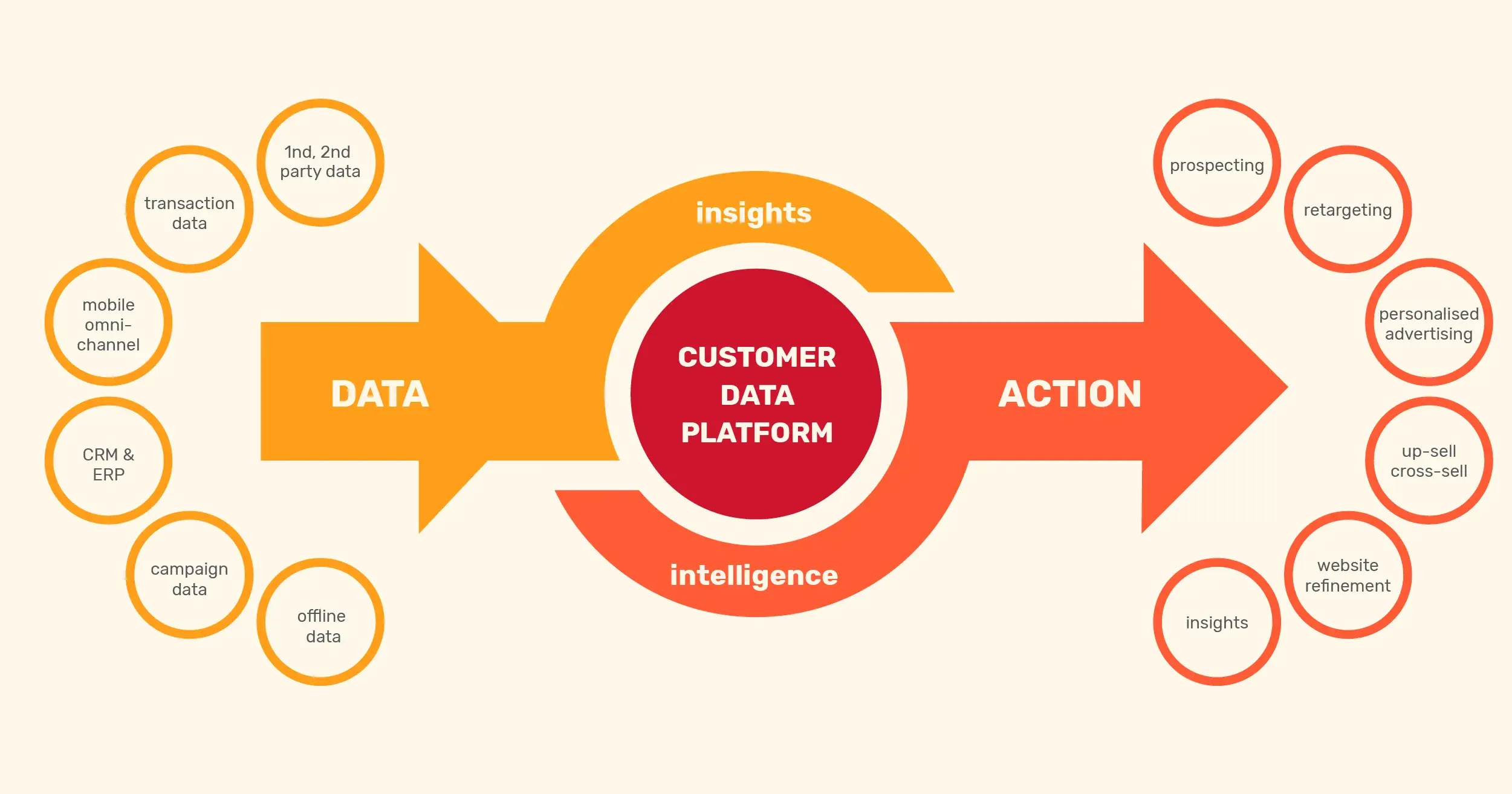
To compare and connect all data and gain valuable insights, you’ll need to collect and integrate it in a unified way through a customer data platform. This approach allows you to deploy machine learning, which adds an extra layer of intelligence – like predictive analytics – and can lead to more efficient actions. The latter includes, for example, targeted recommendations to lead to more engagement.
Unification vs. diversification
A customer data platform allows companies to tackle both data unification and diversity at the same time. This double approach is necessary to get useful benchmarks across sites and departments. While general agreements on taxonomy and guidelines are managed centrally (unification), each local marketing and sales team should translate these to the local context, taking into account experience and cultural, demographic, technological, and other factors – or just ‘common sense’ (diversity). Your Facebook ad campaign won’t be of much use in Eritrea, for example, where 0.06% of the population has an active profile.
However, that doesn’t mean performance can’t be compared across teams, or that there’s nothing that teams in different countries can learn from one another. The idea is that the interface close to the customer needs to be managed locally, while customer data needs to be centralised and unified. In other words, your campaign parameters – taxonomy – should be the same across the board. If this is the case, a CDP allows you to combine everything and link the right data to the right channel. How you apply this data depends, again, on the type of the client, market, and local context you’re facing.
Data taxonomy should be handled centrally, but the actual application needs to be adapted to the local context.
From rule based to intelligence
Another interesting technological development is the shift from rule based to intelligent automation. In the past, whoever was behind the screen would decide what we got to see. Now, machine learning and AI can make these decisions without any human intervention. Although many customer data platforms today are still rule-based, they constitute the perfect foundation for these kind of intelligent applications. For example, when the platform detects that a client is more active on certain channels, it can autonomously decide to prioritise these channels going forward. Many customer data platforms evolve towards a hybrid application that combines a ruled-based approach with a level of automated intelligence.
This kind of self-learning also makes it possible to bypass certain standard assumptions. When someone buys a grill, the logical upselling step might be to advertise some nice juicy steaks. But what if this person has bought meat before, and is – gasp – now a vegetarian? In this case, the algorithm creates a profile of the customer and evaluates every marketing choice in terms of elements from that profile. It’s important to understand, however, that algorithms aren’t infallible. They still require staff supervision and a whole lot of common sense.
Smart algorithms enable us to bypass certain standard assumptions. But it’s important to understand that they are not infallible.
Discover-define-deploy
What we hope is becoming increasingly clear by now is that the specific technology you choose to set up your data pipeline doesn’t matter nearly as much as the underlying principles. At MultiMinds, we adhere to a ‘discover-define-deploy’ methodology, in which we first map the specific goals and needs of an organisation. Only then do we start looking for the right technological components to implement.