How are your customers really feeling? Sentiment analysis dissects the emotions behind written messages
Venture far enough into the rabbit hole that is Twitter, and you’ll find a cesspit of cynical comments, trolls and gloomy outlooks. But of course, not every utterance wears the emotions of the author on its sleeve. Ever wondered what people really feel when writing about your company? Are they just being polite while secretly frustrated? Sentiment analysis is an AI use case that quantifies the emotion behind a written message and that will help you discover the true emotions of your customers.
In short
Sentiment analysis is the analysis of natural language (spoken word or text) to identify the emotions contained in subjective information. In other words: how positive or negative is a message, based on the words used? The point of this analysis is to understand how your users really feel, and deduct why they feel like this.
How it works
Let’s say you work for a railway company, and you’re trying to figure out how satisfied users are by analyzing messages on social media.
Once you’ve scraped all the relevant messages from a platform, the AI algorithm of sentiment analysis will analyze every word, and attribute a score to each one. This score represents how strong the word is, either positive or negative.
By analyzing the whole message and adding up all individual word scores, sentiment analysis can effectively quantify the emotion of the message. So if the morning commute on a certain track is very crowded or suffers delays, you’ll be able to quantify how angry travelers are.
This algorithm can be used for any kind of message, so you can easily analyze what your customers are saying or writing in your helpdesk, complaint email, social media, store reviews etc.
So … what’s it good for?
What do you do with this information? The insights sentiment analysis can give you are of course only useful when it tells you why customers are feeling a certain way, or if it can uncover deeper trends or insights.
We decided to test the algorithm in two different use cases that you could easily implement in your business as well.
Use case 1: the helpdesk priority
For this use case, we analyzed a data stream of messages to a helpdesk. The goal of the sentiment analysis algorithm is to analyze how urgent a message is. If a customer has a standard and non-urgent question, and he or she is not particularly annoyed, they will be forwarded to a chatbot.
If users complain that they haven’t been able to log in for three days, the algorithm detects the sense of urgency or state of panic in the message, and can then prioritize this user and forward them to a person at the helpdesk.
Use case 2: trends in theme park reviews
To test whether sentiment analysis can offer deeper insights, we scraped a large data set of reviews from the Universal Studios theme park. We analyzed 18,000 reviews from the last 3.5 years. Once the algorithm attributed a sentiment score to each message, we put them in a graph to see if any trends arose.
And lo and behold, there is a clear dip in the average sentiment of reviews around the same time each year: the first and last two weeks of the year. Several hypotheses can explain this trend – for instance, this is the time of year when the weather is worse on average, so the experiences are less positive overall. Of course, it is up to the company to further validate hypotheses and take appropriate action.
In the example of the railway company, you could analyze similar big data sets to see what kind of impact strikes have on the average satisfaction of travelers.
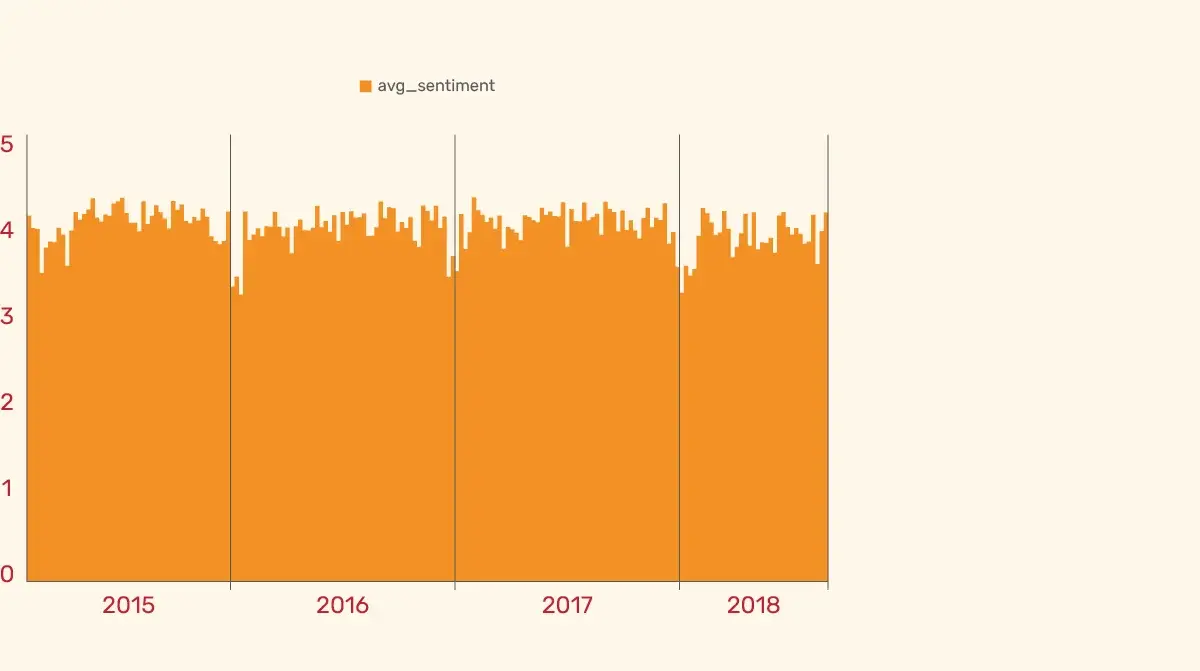
Implement sentiment analysis in your business
These use cases are fictional, though they are based on real datasets. In other words, sentiment analysis can easily be applied to real-life datasets and situations. If your company has any sort of communication from customers, sentiment analysis can offer deeper insights on those messages, allowing you to spot trends and take the right actions to improve satisfaction.
This use case is a proof of concept made by DIAL (data innovation and acceleration lab), using algorithms from the open source Hugging Face project. As part of our “AI & ML lab”, we look at how these technologies can innovate the marketing stack.
Sentiment analysis will soon be a part of our existing dashboard and analysis services. It is particulary useful in cases of social sentiment and customer communication. Keep an eye out for more innovations coming from DIAL!