Beyond the Buzz: predictive analytics
If you could see the future, what would you do with that power? For modern businesses, that dream is slowly but surely becoming a reality, thanks to predictive analytics. Armed with current and historical data and a whole range of analytics technologies, you can make reliable predictions of what’s likely to happen hours, days or even weeks from now. But what’s this buzzword actually about, and what does it mean for you?
The future is certain, most likely
As one of the three major forms of data science, predictive analytics provides important competitive advantages. According to projections by Zion Market Research, the predictive analytics market is set to reach USD 10.95 billion by 2022. And it’s not hard to see why: accurate predictions can help marketing departments to reduce customer churn, tackle supply chain issues before they happen, increase up- and cross selling, and much more.
Of values and probabilities
To make reasonably accurate forecasts, predictive analytics requires lots of data, which is then processed by statistical and/or machine learning technologies to make model-based predictions of future events. The outcome can be specific values or probabilities.
- Value: ‘How many customers will buy my product in the first week after launch?’
- Probability: ‘How likely is this customer to unsubscribe from my services?’
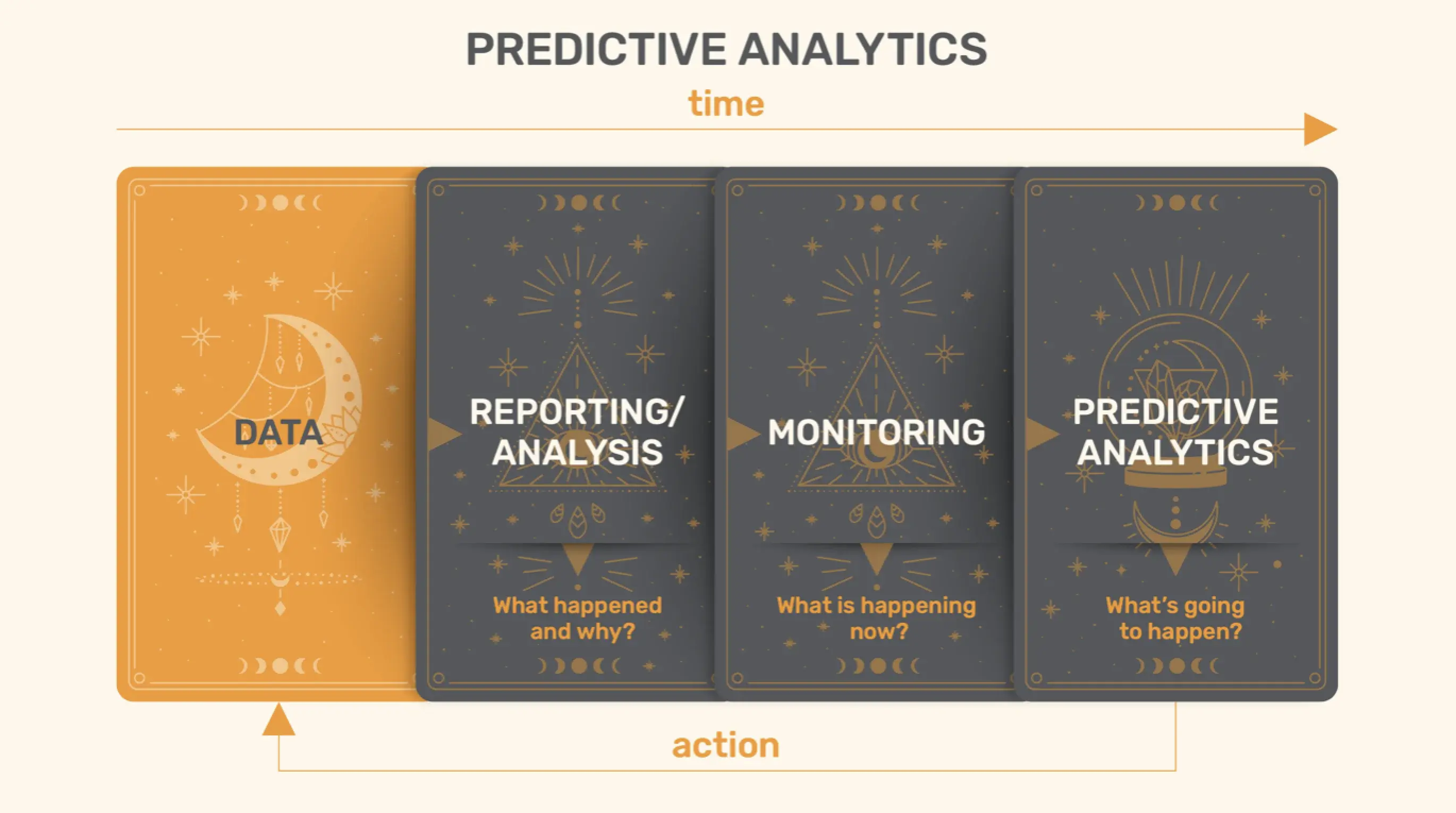
The power of predictions
So what’s the buzz all about? At its core, predictive analytics aims to answer the question: What is (most likely) going to happen based on my current data, and what can I do to prepare for or change that outcome? In the first case, it might mean stepping up production. In the second case, you could make customers at risk of churning reconsider by offering them a well-timed, personalized promotion.
Predictive analytics enable us to prepare for or change what is likely to happen.
Predictive analytics: misconceptions, hurdles and controversies
As promising as this all sounds, there are still a couple of fundamental misunderstandings, hurdles and mild controversies around predictive analytics. We’ll tackle a few below:
- A lot of predictive analytics is descriptive analytics in disguise. Many companies are actually just looking at data after the fact to find out what has happened in the past – for example: how much money did we lose due to the Covid-19 restrictions, what were our most valuable customers last year, etc. These insights can be highly valuable as well, but they don’t tell you anything about the future.
- Accurate predictions require lots of data and not every company or product has the amount of customer interactions needed to make that possible. One-off transactions, for example, like a new floor or a new car, where customer interactions are highly infrequent, make accurate predictions a lot more complex.
- We overfocus on online data when making predictions, giving businesses an incomplete picture of what’s going on. To get a comprehensive view, you’ll need to track offline touch-points too: in-store experiences, helpdesk interactions, …
- Predictions often clash with assumptions and intuitions, especially in the marketing department. Trusting the data model often requires putting your ego aside and taking a broader view.
- ‘Big data’ is being replaced with ‘fast data’. While predictive analytics still requires a large amount of data, the rise of cheap (cloud) computing power makes it possible to make calculations in real-time, based on ‘new’ data.